John Anderton: "I need your help. You contain information. I need to know how to get at it."
In the recent science-fiction thriller "Minority Report," Tom Cruise plays Detective John Anderton who solves future crimes by being immersed in a "data cave," where he rapidly accesses all the relevant information about the identity, location and associates of the potential victim.
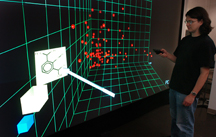
A team at PurdueUniversity is currently developing a similar
"data-rich" environment for scientific discovery that uses high-performance computing and artificial intelligence software to display information and interact with researchers in the language of their specific disciplines.
"If you were a chemist, you could walk right up to this display and move molecules and atoms around to see how the changes would affect a formulation or a material's properties," said James Caruthers, a professor of chemical engineering at Purdue. The method represents a fundamental shift from more conventional techniques in computer-aided scientific discovery.
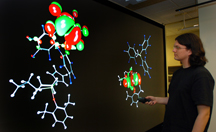
"Most current approaches to computer-aided discovery center on mining data in a process that assumes there is a nugget of gold that needs to be found in a sea of irrelevant information," Caruthers said. "This data-mining approach is appropriate for some scientific discovery problems, but scientific understanding often proceeds through a different method, a 'knowledge discovery' approach.
"Instead of mining for a nugget of gold, knowledge discovery is more like sifting through a warehouse filled with small gears, levers, etc., none of which is particularly valuable by itself. After appropriate assembly, however, a Rolex watch emerges from the disparate parts."
A team of researchers at Purdue led by Caruthers is developing a computer environment that allows experts to talk naturally in their specific scientific language. That way, the researchers don't have to deal with computerese and can take full advantage of the most advanced visualization capabilities to become more engaged in the scientific discovery process, Caruthers said.
Such a system could become crucial for enabling scientists to deal with the recent explosion of data now available to them. The source of this flood of data is "high-throughput" experimentation, in which hundreds or thousands of experiments are conducted simultaneously in tiny vessels that are sometimes as small as a few human hairs. Having so much information presents a challenge: it is difficult for researchers to find what they are looking for within this huge sea of data.
Drowning in data, yet starved of information - Ruth Stanat in The Intelligent Organization
"You run the risk of drowning in data," said W. Nicholas Delgass, a Purdue professor of chemical engineering. "What you really want is
knowledge, not data."
Purdue researchers believe they have a solution to the problem. They are developing a method to extract knowledge from data, promising to speed up the process of discovery in many areas of research, including work aimed at creating new drugs, fuel additives, catalysts and rubber compounds.
The method, called
discovery informatics, enables researchers to test new theories on the fly and literally see how well their concepts might work in real time via a three-dimensional display, said Venkat Venkatasubramanian, another professor of chemical engineering working to develop the new system.
Discovery informatics depends on a two-part repeating cycle made up of a "forward model" and an "inverse process" and two types of artificial intelligence software: hybrid neural networks and genetic algorithms.
The forward model combines fundamental knowledge and rules of thumb with neural networks � software that mimics how the human brain thinks � to tell researchers how a particular material will perform.
The inverse process is just the opposite: Researchers enter the properties they are looking for, and the system gives them a molecular structure or formulation that will likely have those properties. The inverse process cannot begin until the forward model is completed because the former depends on information in the model.
No comments:
Post a Comment